Latest News
Data science and the personalised medicine revolution
16 Aug, 20245 minutesData science has revolutionised the life science field in recent years, pushing the boundari...
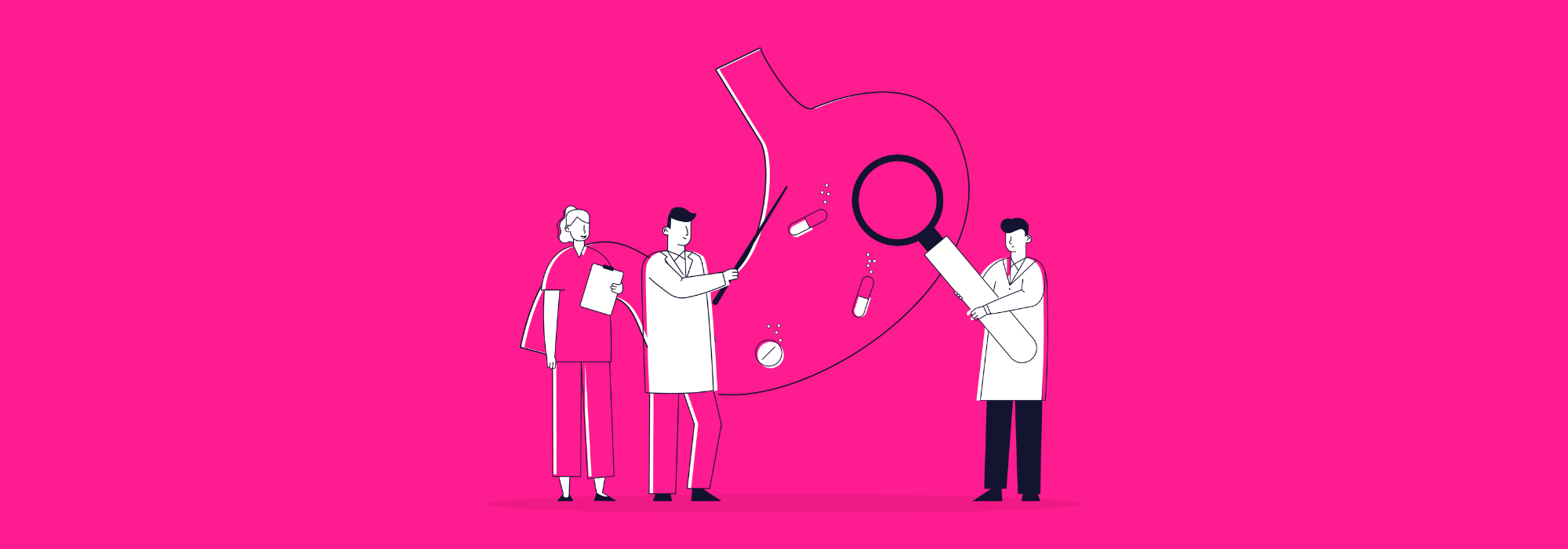
Data science has revolutionised the life science field in recent years, pushing the boundaries of what is possible in precision medicine. Data-driven approaches are helping us to predict, prevent, and treat diseases more effectively than ever before.
Data has become the lifeblood of modern medicine, with vast amounts of health-related information pouring in from diverse sources like electronic health records, genomics data, and wearables. RBC Capital Market projects that healthcare data is estimated to grow at a compound annual growth rate of 36% through to 2025. This explosion in data availability is enabling a more profound understanding of individual patients, which, in turn, is paving the way for tailored therapies and targeted treatments.
Machine Learning: The workhorse of precision medicine
Machine learning (ML), a subset of artificial intelligence (AI), has emerged as a game-changer in the life science sector, particularly in precision medicine. It allows computers to learn from vast data sets and identify patterns that would be impossible for humans to detect. In a recent study, researchers from Google and Verily Life Sciences demonstrated that machine learning algorithms could predict cardiovascular risk factors more accurately than traditional statistical methods.
A prime example of machine learning in precision medicine is the Human Cell Atlas project. This ambitious initiative aims to create comprehensive reference maps of all human cells, providing a fundamental resource for understanding human health and disease. By combining large-scale data collection, advanced bioinformatics, and machine learning algorithms, the Human Cell Atlas project is working to uncover the unique molecular signatures of individual cells and their roles in various diseases. This detailed knowledge has the potential to transform our understanding of disease mechanisms and facilitate the development of highly targeted therapies, ultimately revolutionising precision medicine.
Genomics and bioinformatics: personalising the genetic blueprint
Genomics and bioinformatics are at the heart of personalised medicine, driving significant advancements in our understanding of diseases and potential treatments.
One of the most prominent examples of personalised medicine is in cancer treatment, where genomics and bioinformatics have enabled precision oncology. By sequencing tumour genomes, researchers can identify the specific genetic alterations driving a patient's cancer, allowing for targeted therapies that address the underlying molecular mechanisms.
Pharmacogenomics, the study of how a person's genetic makeup influences their response to drugs, is another prime example of personalised medicine in action. By analysing an individual's genetic information, clinicians can make more informed decisions about drug prescriptions, dosing, and potential adverse effects. For instance, research has shown that the anticoagulant drug warfarin's effectiveness is influenced by variations in the genes CYP2C9 and VKORC1, which affect the drug's metabolism and target, respectively. Genotyping these genes before prescribing warfarin can help optimise dosing and minimise the risk of adverse reactions.
Digital Twins: Simulating the Human Body for Personalised Treatments
Digital twins, virtual replicas of physical objects, are gaining traction in the life science sector as a powerful tool for personalised medicine. By simulating an individual patient's anatomy and physiology, digital twins can help predict how a person might respond to a specific treatment, enabling clinicians to tailor therapies more precisely. Companies like GE Healthcare and Dassault Systèmes are leading the way in developing sophisticated digital twin platforms for healthcare applications.
A clear example of digital twins in action is the Living Heart Project, an initiative led by Dassault Systèmes. This project aims to create a highly accurate, 3D digital twin of the human heart, allowing researchers and medical professionals to explore and test various treatment options in a simulated environment before applying them to a real patient. By simulating blood flow, electrical activity, and other physiological processes, the Living Heart Project can help identify potential risks and benefits of specific treatments, such as the optimal placement of a pacemaker or the effect of a new drug on heart function.
Another example is the work of Wolfgang Wall, a professor of computational mechanics at the Technical University of Munich, who has been developing digital twin models of lungs to simulate the effects of various inhaled medications on patients with respiratory diseases like asthma and chronic obstructive pulmonary disease. The eventual goal is to provide an individual lung model for each patient on a ventilator and optimise its use, ultimately leading to more personalised treatment plans.
Challenges and Opportunities
While the personalised medicine revolution has made remarkable strides in recent years, there are still numerous challenges to overcome.
- Data Privacy and Security: Safeguarding sensitive health data is vital for maintaining public trust. Solutions include encryption, access controls, and regulatory compliance.
- Data Integration and Interoperability: Standardisation and harmonisation of diverse data types and formats are essential for seamless data exchange.
- Scalability and Computational Power: Investments in high-performance computing infrastructure and cloud-based storage are crucial for handling the data influx.
- Regulatory and Ethical Considerations: Ensuring the safe and responsible use of AI-driven tools and addressing health disparities is important to prevent exacerbating existing inequalities.
Despite these hurdles, the opportunities for data science in personalised medicine are immense.
- Early Detection and Prevention: Data science can help identify early disease indicators, allowing for targeted preventive measures.
- Improved Diagnostic Accuracy: Machine learning algorithms can increase diagnostic accuracy, leading to better patient outcomes.
- Tailored Treatment Plans: Personalised medicine can develop targeted treatment plans based on an individual's unique factors, resulting in fewer side effects.
- Drug Development and Repurposing: Data science can accelerate drug development and repurpose existing drugs, saving time and resources.
- Enhanced Patient Engagement: Patient-generated data can empower patients to manage their health, leading to better treatment adherence.
As more data becomes available and computational power continues to increase, the potential for breakthroughs in disease prevention, diagnosis, and treatment is enormous. By investing in the right talent and fostering a culture of innovation, companies in the life science sector can contribute to this revolution and make a lasting impact on global health.
The future is personalised
The personalised medicine revolution, driven by data science, is transforming the life science sector and redefining the way we approach disease prevention, diagnosis, and treatment. By embracing the power of big data, machine learning, genomics, and digital twins, while investing in talent, fostering a culture of innovation, and promoting collaboration, we can unlock the potential of precision medicine and help pave the way for a new era of personalised healthcare.