Latest News
The cost of poor data quality in drug development
17 May, 20223 minutesData is integral to the development of new drugs. As the linchpin around which analysis, sub...
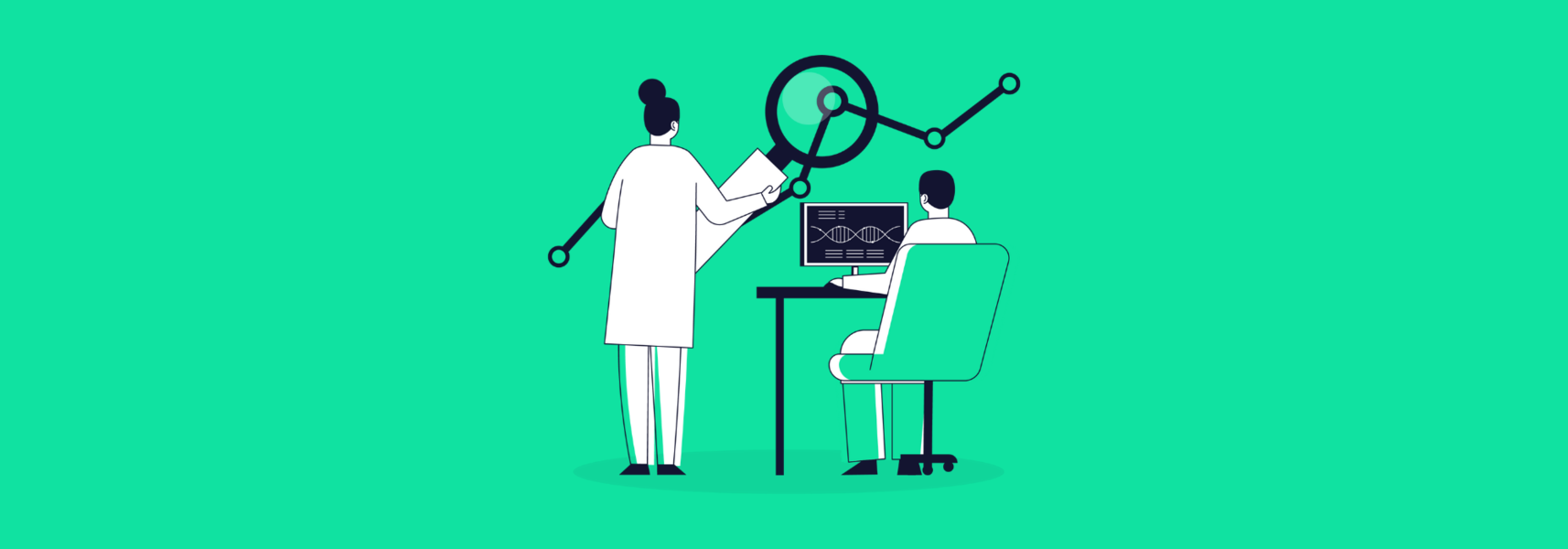
Data is integral to the development of new drugs. As the linchpin around which analysis, submission, approval, and marketing of a new product revolves, it’s one of the most valuable assets at the disposal of drug development companies – but only when its integrity can be relied upon.
Data has always been key to the discovery, research, and development of a new drug. But it holds particular relevance in today’s pharmaceutical landscape due to the increased interest in artificial intelligence, as well as other process-enhancing technologies that rely on enriched data.
Promising early use of these technologies give a glimpse into a future where data and technology work in synergy and the outcome is a more streamlined and cost-effective route to market. The reality is, though, that such technology is rendered unusable without accurate and up-to-date data to work with.
Effective management, processing, and integration of data from development through to clinical trials and beyond is crucial for advancing medicine, ensuring treatments are applicable to a real-world population, and for protecting an organisation’s bottom line.
The poor-quality data snowball effect
When data quality isn’t up to scratch, there’s a knock-on effect in any later stages of the drug development process. When data of inadequate quality passes through trial monitoring stages it can lead to problems at approval stage, meaning competitors take the lead, major delays to market are incurred for the treatment, and the organisation(s) involved face potential damage to their reputation too.
Early last year US-based pharma Zogenix faced its second FDA application denial for their drug Fintepla on the grounds that datasets from their clinical trials were missing key information. It came after an initial rejection for the same drug back in 2019, with the FDA citing an ‘incorrect version of the clinical dataset’.
Both rejections highlight the importance of effective data collection and management, particularly at trial stage, and how a poor data strategy and failure to recognise errors can stop the development process in its tracks and prove costly for the organisations involved.
Factors that can impact data quality
From discovery through to clinical development and beyond, the scope for incorrect or mismanaged data sets is wide.
The most common factor that can negatively impact data cleanliness is manual error. During data entry, typographical, copying or coding errors can occur, which is why roles such as clinical trial monitors are so essential to ensuring reported data is accurate.
Aside from inputting errors, adhering to local laws can present a challenge for the methods of collecting and storing data, as organisations conducting research across multiple countries adapt to potential differences in data regulations. Failure to understand regulatory requirements, as well as limits on the types of data you can collect in certain geographies, can cause dispersed data and lead to inaccurate analyses.
Poor trial design with inconsistent endpoints can also impact data quality, as can staff or project team turnover.
The benefits of good data hygiene
A solid data collection, management, and processing infrastructure is paramount for gathering strong evidence for submission and negating the need for ‘rescue studies’ which can add hundreds of thousands in additional costs to the drug development process.
But understanding the value of data health can also allow for organisations to invest in the technology to process and apply it, which can streamline processes and offer an edge on any competitors in the space. Organisations are clamouring to implement new digital strategies like AI, machine learning, personalised medicine, and automation, all of which rely heavily on data integrity.
Involving the right people at the right time is key to a successful data strategy; clinical trial monitors are essential throughout trial phases, and involving biostatisticians and statisticians during each stage of the development cycle will strengthen protocol development, data management, monitoring and reporting capabilities. Statisticians will also be able to advise on trial design methods, and alleviate problems that might occur as a result of trial design.