Latest News
How can we encourage collaboration in data science teams?
18 Apr, 20238 minutes86% of employees and executives state that a lack of effective collaboration is the main cau...
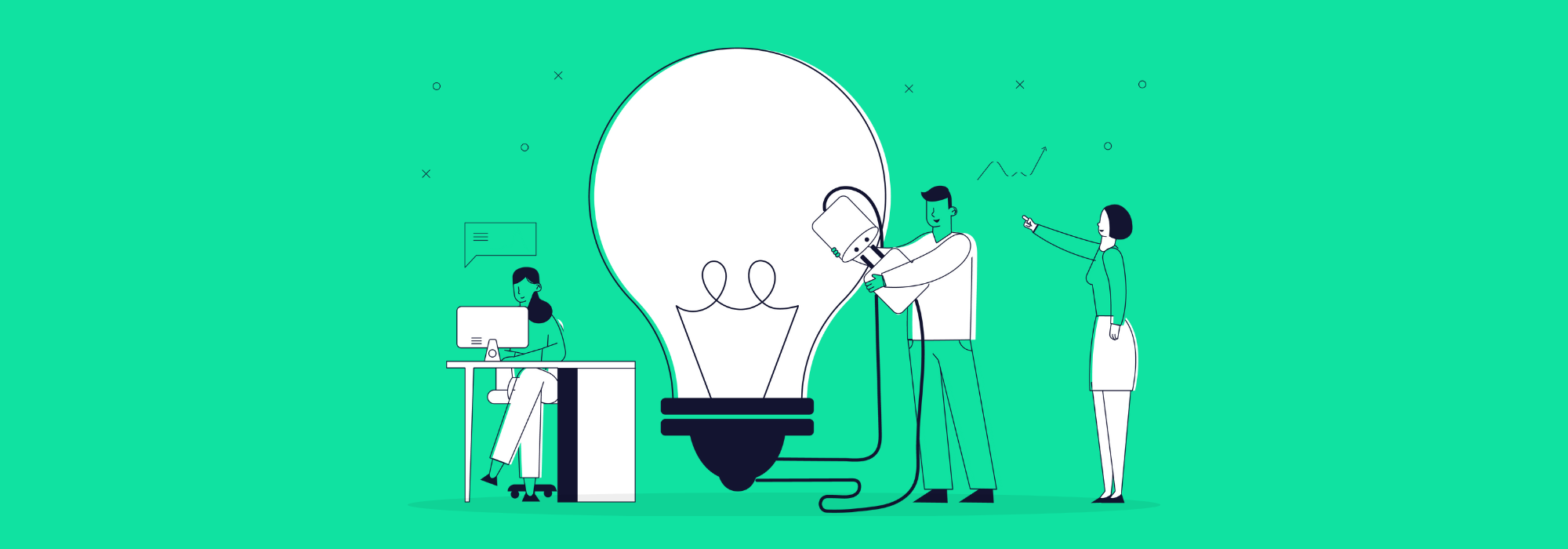
86% of employees and executives state that a lack of effective collaboration is the main cause of workplace failures. This is no different in your data science department. A collaborative environment not only improves the quality of work but also ensures that different perspectives are brought together, leading to more comprehensive and innovative solutions, all essential to harness the full potential of data science and maintain a competitive edge.
However, creating this type of environment is easier said than done and with the position of data scientist being considered one of the most remote-friendly jobs, encouraging collaboration within your data science team is no mean feat. Here are five strategies that you can implement to enhance collaboration within your data science department.
Encourage Cross-functional Collaboration
Data scientists should be encouraged to work closely with colleagues from other departments, such as biology, chemistry, and clinical research. This interdisciplinary approach can lead to better insights and solutions, as well as increase team engagement. According to McKinsey, cross-functional collaboration can result in a 30% improvement in team performance and innovation.
One way that leaders can facilitate cross-functional collaboration is by creating joint projects or initiatives that require input from multiple departments. For instance, leaders can assign a data science team to collaborate with clinical research teams on developing predictive models for patient outcomes. By providing a common goal, this joint effort will encourage team members to share their expertise and learn from each other, ultimately enhancing collaboration.
Another way is through organising regular team meetings and workshops that involve data scientists and other departments. These events can provide opportunities for team members to discuss ongoing projects, brainstorm solutions, and share their expertise with colleagues from different backgrounds. For instance, leaders can schedule biweekly or monthly meetings where data scientists present their latest findings and solicit feedback from colleagues in other departments, such as clinical research or drug development.
Implement Agile Methodologies
Adopting Agile methodologies can help streamline project management and enhance team collaboration. Agile principles, such as iterative development and continuous feedback, allow data scientists to work more efficiently and adapt to changing requirements quickly. It’s been found that Agile projects have a 64% success rate, whereas projects under the competing methodology known as waterfall only have a 49% success rate. And with companies experiencing a 60% growth in revenue and profit after adopting Agile, this is vital for every business to implement.
Some life sciences companies have successfully implemented Agile methodologies in their data science teams. For example, the UK branch of Novartis has adopted Agile principles to create a more flexible and collaborative environment for its team as a whole. The leadership team found:
- Delivery time – reduced by half compared to internal benchmarks.
- Production of materials – 70% reduction in time spent in approval, and costs were 50% lower than expected.
- Team satisfaction – rated highly, with confidence to repeat this achievement in future work at +90%.
To implement Agile methodologies in data science teams, leaders can start by adopting Scrum or Kanban frameworks. For example, they can organize the team into smaller, cross-functional units and assign a Scrum Master or Agile Coach to guide the process. Daily stand-up meetings can be introduced to ensure that team members are aligned, and progress is continually tracked through visual management tools like task boards. Regular sprint reviews and retrospectives can also be held to evaluate progress, address challenges, and continuously improve the team's processes.
Create a Culture of Knowledge Sharing
Encouraging a culture of knowledge sharing can improve team collaboration, as well as enhance the skillset of individual data scientists. This can be achieved through regular presentations, workshops, and internal publications. Companies that promote knowledge sharing can see a 25% increase in productivity, according to McKinsey.
In the life sciences sector, companies such as Roche have developed platforms for data scientists to share their expertise and learn from others. This includes internal forums and workshops, as well as collaboration with external partners.
For example, during the COVID-19 pandemic, Roche Canada assembled the Data Science Coalition, a group of like-minded public and private organisations, whose purpose was to bring actionable COVID-19 intelligence to patients, frontline healthcare providers, institutions, supply chains and governments around the globe. The Coalition can gather and process large amounts of global information quickly. They then provide it back in formats that are easy for scientists, epidemiologists, health agencies, statisticians, governments, and others to understand and use. The Coalition's teams created a worldwide report to aid governments and healthcare providers in handling and getting ready for patients, identifying community risks, and planning for the future.
Leverage Collaborative Tools and Platforms
Utilising collaborative tools and platforms can greatly enhance the efficiency and effectiveness of data science teams. These tools facilitate communication, project management, and documentation, ultimately leading to improved collaboration. With 72% of data scientists preferring to work remotely, encouraging collaboration is vital. McKinsey’s study about the future of remote work agrees with this statement, having found that employees who feel more included in workplace communication are almost 5 times more likely to report increased productivity.
A popular collaborative platform that leaders can implement for their data science teams is that of Jupyter Notebooks. This is an open-source platform that allows data scientists to create and share live code, equations, visualizations, and narrative text. This tool enables seamless sharing and collaboration on data science projects.
Recognise and Reward Team Success
Recognising and rewarding team success can lead to higher levels of engagement and collaboration. By celebrating collective achievements, organisations can create a positive and inclusive work environment where data scientists feel valued and motivated to contribute. A survey by Gallup found that 66% of employees agree when the whole team are praised, the quality of their work increases. It’s also been found that when rewards are given only for individual achievements, employees tend to work alone instead of collaborating with others. On the other hand, when teams are rewarded for their collective achievements, such as with bonuses, company benefits, career opportunities, or recognition, they are more motivated to work together towards their goals
Fostering collaboration within data science teams is crucial for driving innovation and success in the life sciences industry. By implementing strategies such as cross-functional collaboration, Agile methodologies, knowledge sharing, mentorship, collaborative tools, and recognition programs, organisations can unlock the full potential of their data science teams. With a collaborative and engaged team, companies in the life sciences sector can harness the power of data-driven decision-making to improve patient outcomes, accelerate research, and create a brighter future for all.